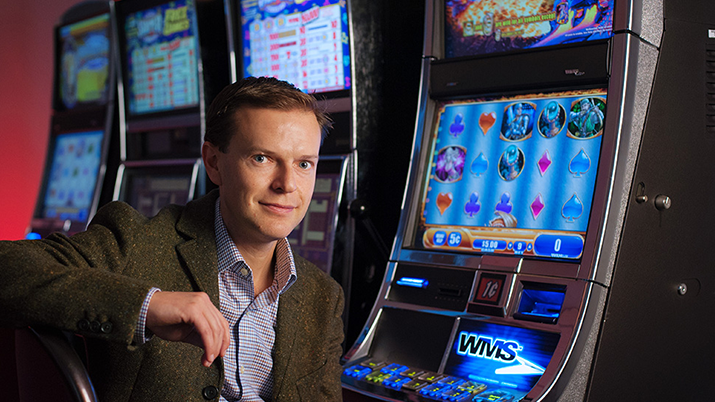
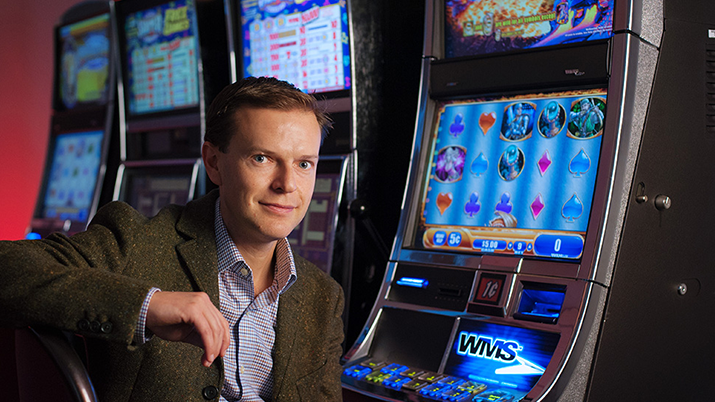
Luke Clark at UBC’s Centre for Gambling Research
Researchers at the Centre for Gambling Research at UBC have successfully used machine learning to identify online gamblers whose betting behaviour could indicate a gambling problem.
By analyzing data from actual bets placed on the British Columbia Lottery Corporation’s (BCLC) PlayNow.com platform, an algorithm developed by the Centre for Gambling Research at UBC predicted with approximately 75-per-cent performance which players had, at some point, tried to curb their gambling by enrolling in BCLC’s Voluntary Self-Exclusion program (VSE).
The results show that algorithms tracking the behaviour of online gamblers could allow responsible operators to intervene before customers develop serious problems.
“The ultimate goal here is to predict risk before someone is actually displaying problems on the website. We’d like to be able to anticipate risk before things reach that extreme end. There’s a lot of further work to do in this vein, but I think this is encouraging as a proof of principle.”
“The ultimate goal here is to predict risk before someone is actually displaying problems on the website,” said Dr. Luke Clark, senior author of the study and director of the CGR. “We’d like to be able to anticipate risk before things reach that extreme end. There’s a lot of further work to do in this vein, but I think this is encouraging as a proof of principle.”
Players categorized into two groups
BCLC provided the research team with data on more than 575 million bets placed on the PlayNow.com platform from October 2014 to September 2015. The players were anonymous, but the research team could see which ones had a history of enrollment in the self-exclusion program.
The self-exclusion program is designed to help individuals who are having difficulties associated with gambling. Participants sign up when they need to take time away from gambling. BCLC helps them honour that commitment. In the case of PlayNow.com, those enrolled in the self-exclusion program have their accounts blocked for the duration of their VSE term.
Of the 19,683 players included in this study, 2,157 had been in the program.
The UBC researchers trained their algorithm by allowing it to analyze betting patterns of players who were categorized either as self-excluders or not. Ten per cent of the data was set aside from the training process so it could be used later to test the algorithm’s performance. The model could tell with about 75-per-cent performance which betting patterns matched the profile of a self-excluder. A statistical model making random choices would achieve accuracy of around 50 per cent.
Biggest signal came from variance in money bet per session
The researchers also learned which behaviours the model was relying on to make its predictions. Of the 20 measures that the researchers used as inputs—including number of bets per day, total net win, and the number of different games played in a session—the variability in the amount bet per session turned out to be the most significant indicator.
For whatever reason, self-excluders had a tendency toward large swings in money wagered from session to session.
“It’s not necessarily a high average bet overall, which you might expect, but it’s a more variable pattern which is predictive,” said Clark, who is a professor in UBC’s department of psychology. “We think that is telling us something about loss chasing, which is a key feature in people with gambling problems. Our next question in this work is to devise some better measures of chasing within this kind of data.”
Loss chasing is when a gambler either continues gambling, or in some cases even goes back another day, to try to recover their losses.
How it could be put into practice
Online gaming allows for a level of behaviour tracking that would be nearly impossible in a land-based casino environment. Clark credits BCLC for sharing the data that made this study possible, since researchers have little access to real-world gambling data, most of which has come from Europe. BCLC provided the data because the research complements other work the organization is doing to predict and prevent gambling-related harm.
The UBC algorithm was built using only a fraction of the data that would be available to online gambling operators who choose to employ machine learning in the future. Those algorithms would learn more with every bet placed, and could also have access to a range of other information about players for more accurate profiling.
While the UBC study shows that the approach can work, ongoing access to greater volumes of data would lead to higher accuracy and allow more gamblers to get the help they need when they need it.
The study was published today in International Gambling Studies.
This news release was originally posted on the UBC News website.